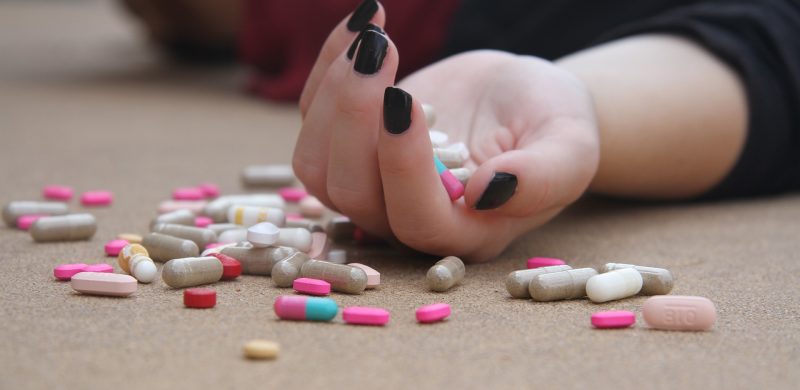
Scientists have trained a computer program to identify people with suicidal thoughts based on their brain scans. The method could one day be used for diagnosing mental health conditions, researchers say.
Nearly a million people worldwide die by committing suicide every year, and predicting suicide remains difficult, especially because many people feel uncomfortable talking about the issue. In a study published by the journal Nature Communications, researchers observed the brain activity of two groups of adults — one who had suicidal thoughts and one who didn’t — while they thought about words such as “evil” or “praise.” They fed this data to an algorithm that learned to predict who had suicidal thoughts with 91 percent accuracy. It also predicted whether someone had attempted suicide before with 94 percent accuracy.
The algorithm isn’t perfect and a medical test would have to be. It may also not become widely used since brain scans are expensive. But “it’d be nice to have this additional method,” says study author Marcel Just, a psychologist at Carnegie Mellon University.
Thirty-four volunteers participated in the study: 17 with suicidal thoughts and 17 without. The volunteers read 30 words that were either positive (“bliss”), negative (“cruelty”), or related to death (“suicide”) and thought about the meanings while undergoing a type of brain scan called fMRI.
Whenever we think about a given subject, our neurons fire in a specific way, says Just. Your neurons might fire in one pattern for the word “hammer,” for example, and in another pattern for “dog.” Measuring patterns like this is more accurate than other brain studies that only look at the general brain region that is activated.
Researchers found that the responses to six words — “death,” “trouble,” “carefree,” “good,” “praise,” and “cruelty” — showed the biggest differences between the two groups of participants. So, they gave a machine-learning algorithm these results for every person except one. For any given word, they told the program which neural activation patterns came from which group. Then, they gave them the missing person’s results and asked the algorithm to predict which group the person belonged to. The machine got it right 91 percent of the time. In a second experiment, scientists used the same methods to teach an algorithm to distinguish people who had attempted suicide from those who hadn’t, this time with 94 percent accuracy.
Blake Richards, a neuroscientist at the University of Toronto, says the results are interesting, but may not be strong enough to make the test useful for diagnosis. And the activity patterns are still correlation, not causation. “There is undoubtedly a biological basis for whether someone is going to commit suicide,” he says. “There’s a biological basis for every aspect of our mental lives, but the question is whether the biological basis for these things are sufficiently accessible by fMRI to really develop a reliable test that you could use in a clinical setting.” The accuracy of the results may be high, but for the program to be useful in a clinical setting, and to justify any type of medical intervention, it would need to be basically perfect, he says.
Just acknowledges that the small number of participants is a limitation of today’s research. Still, he believes that in the future the algorithm could be used to diagnose people with suicidal thoughts, or even to check whether treatments for psychiatric disorders are working. To improve the accuracy of the algorithm, he’d like to do more research with more volunteers, and try to distinguish between people who have been diagnosed with specific psychiatric disorders.
It’s difficult to identify whether someone is feeling suicidal by asking. According to studies, roughly 80 percent of those who go through with the act denied feeling suicidal the last time they spoke to a mental health care expert. However, AI-related technology which is also being applied to things like spotting cancerous cells might offer a way to identify these thoughts and prompt a timely intervention. Researchers have developed a machine learning algorithm that identifies suicidal tendencies based on activity in specific brain regions.
In the study, the researchers started with 17 young adults between the ages of 18 and 30 who had recently reported suicidal ideation to their therapists. Then they recruited 17 neurotypical control participants and put them each inside an fMRI scanner. While inside the tube, subjects saw a random series of 30 words. Ten were generally positive, 10 were generally negative, and 10 were specifically associated with death and suicide. Then researchers asked the subjects to think about each word for three seconds as it showed up on a screen in front of them. “What does ‘trouble’ mean for you?” “What about ‘carefree,’ what’s the key concept there?” For each word, the researchers recorded the subjects’ cerebral blood flow to find out which parts of their brains seemed to be at work.
But that’s where this is all headed. Right now, the only way doctors can know if a patient is thinking of harming themselves is if they report it to a therapist, and many don’t. In a study of people who committed suicide either in the hospital or immediately following discharge, nearly 80 percent denied thinking about it to the last mental healthcare professional they saw. So, there is a real need for better predictive tools. And a real opportunity for AI to fill that void. But probably not with fMRI data.
It’s just not practical. The scans can cost a few thousand dollars, and insurers only cover them if there is a valid clinical reason to do so. That is, if a doctor thinks the only way to diagnose what’s wrong with you is to stick you in a giant magnet. While plenty of neuroscience papers make use of fMRI, in the clinic, the imaging procedure is reserved for very rare cases. Most hospitals aren’t equipped with the machinery, for that very reason. Which is why Just is planning to replicate the study—but with patients wearing electronic sensors on their head while they’re in the tube. Electroencephalograms, or EEGs, are one hundredth the price of fMRI equipment. The idea is to tie predictive brain scan signals to corresponding EEG readouts, so that doctors can use the much cheaper test to identify high-risk patients.
Other scientists are already mining more accessible kinds of data to find telltale signatures of impending suicide. Researchers at Florida State and Vanderbilt recently trained a machine learning algorithm on 3,250 electronic medical records for people who had attempted suicide sometime in the last 20 years. It identifies people not by their brain activity patterns, but by things like age, sex, prescriptions, and medical history. And it correctly predicts future suicide attempts about 85 percent of the time.
“As a practicing doctor, none of those things on their own might pop out to me, but the computer can spot which combinations of features are predictive of suicide risk,” says Colin Walsh, an internist and clinical informatician at Vanderbilt who’s working to turn the algorithm he helped develop into a monitoring tool doctors and other healthcare professionals in Nashville can use to keep tabs on patients. “To actually get used it’s got to revolve around data that’s already routinely collected. No new tests. No new imaging studies. We’re looking at medical records because that’s where so much medical care is already delivered.”
And others are mining data even further upstream. Public health researchers are poring over Google searches for evidence of upticks in suicidal ideation. Facebook is scanning users’ wall posts and live videos for combinations of words that suggest a risk of self-harm. The VA is currently piloting an app that passively picks up vocal cues that can signal depression and mood swings. Verily is looking for similar biomarkers in smart watches and blood draws. The goal for all these efforts is to reach people where they are—on the internet and social media—instead of waiting for them to walk through a hospital door or hop in an fMRI tube.